Prediction of peptide properties for proteomics
Course outline and goals
This course focuses on the application of machine learning to predict different peptide properties (regression tasks) that are relevant to mass spectrometry-based proteomics [1].
During this course, independent of the assigned prediction task, students are going to:
- perform literature research of a pre-defined topic
- get a general understanding of machine learning and how to apply machine learning to biological data [2,3]
- develop and correctly evaluate a machine learning model including parameter optimization [2,3]
- present milestones and final results in various presentations to the other students and supervisors
- summarize results in a paper-like scientific report at the end of the course
- gain a first look at the peer-review process by critically evaluating the reports of other students
Students will work in groups of ~3. They will present their topic, background as well as their datasets together. They will work on the same prediction task, but will follow different approaches as discussed with their supervisor. In the end, each group will merge their result, present them and and final conclusion in a talk and a written scientific report.
Course summary
Type | 5SWS (1 year module, 2 SWS/Summer, 3 SWS/Winter) |
---|---|
ECTS | 9 |
Lecturer | Mathias Wilhelm, Wassim Gabriel, Ludwig Lautenbacher |
Time | Monday, 14:00 - 16:00 (Can be adjusted based on student feedback) |
Location | Freising/Weihenstephan |
Language | English |
Kick-off meeting
The kick-off meeting is scheduled to take place in-person on Monday, 24.04, 14:00-16:00, but may be moved in consultation with the participants. Attendance is mandatory.
All important information including detailed description of the topics will be made available via Moodle beforehand.
Timeline
Date | Type | Topic |
---|---|---|
2023-04-24 | Lecture | Kickoff + Intro to Mass Spectrometry + Topic Introduction |
2023-05-08 | Lecture | Literature research |
2023-05-15 | Lecture | Introduction to machine learning |
2023-05-22 | Lecture | Scientific presentations |
2023-06-02 | Student presentation | Introduction talks |
2023-06-05 | Submission | Introduction report |
2023-06-26 | Student presentation | First milestone talks |
2023-07-14 | Student presentation | Progress report + Q&A |
2023-10-09 | Student presentation | Second milestone talks |
2023-10-16 | Lecture | Scientific writing and peer review process |
2023-11-06 | Submission | Scientific report (draft) |
2023-11-20 | Submission | Peer-review revision |
2024-01-08 | Student presentation | Final presentation |
2024-01-15 | Submission | Scientific report (final) |
Topics
Each topic will be worked on by 3-4 students.
Prediction of collisional-cross-section
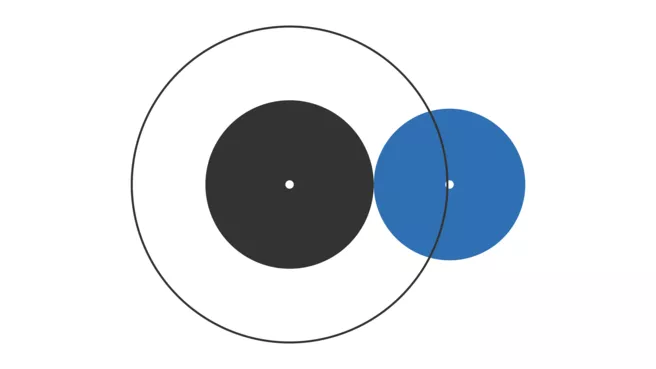
Prediction of retention time
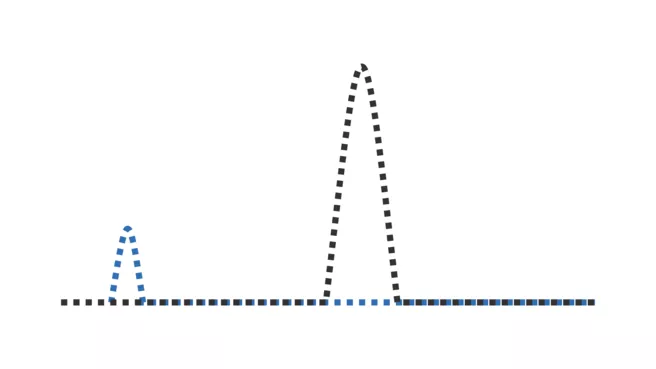
Prediction of compensation-voltage
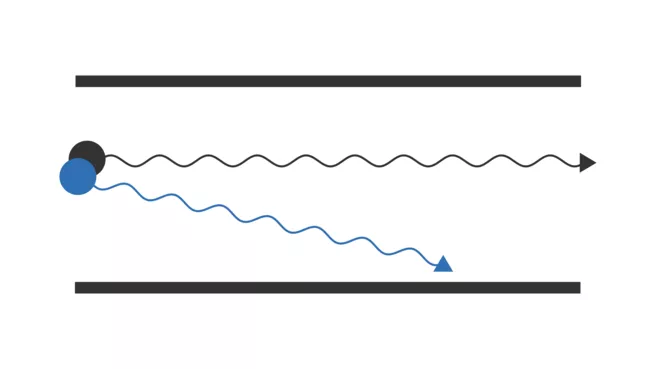
Prediction of fragment intensities
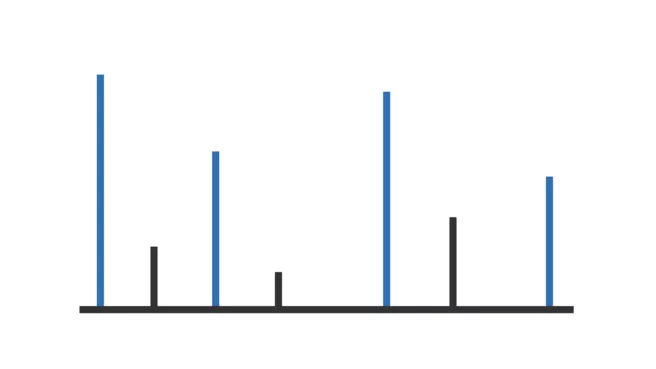