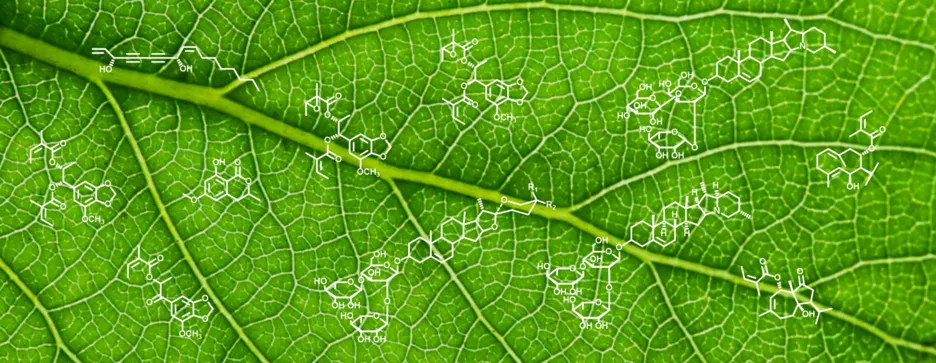
PHYTOMETABOLOMICS
From plant stress to metabolic response
Plants collectively synthesize a wide variety of structurally diverse secondary metabolites that are of immense importance for the growth and development of plants and constitute an arsenal of chemical defenses. These molecules are biosynthesized, upregulated, or both under specific abiotic stresses and pathogenic attacks and therefore provide survival tactics for plants. The science-driven breeding of stress-tolerant cultivated plants, which would allow for a reduction in harvest losses and prevent an undesirable decrease in quality attributes, requires a new quality of knowledge regarding the molecular markers associated with relevant agronomic traits, the quantitative metabolic responses of plants to stress challenges, and the mechanisms controlling biosynthesis. To characterize and identify the marker and bioactive metabolites upregulated during plant stress and to discover the link between stress resistance and metabolites, the concept of phytometabolomics has been developed by the group of Prof. Dr. Corinna Dawid. This concept comprises a minimum of nine steps (see Figure 1; taken from Dawid, 2021). This research will help to navigate breeding programs and optimize post-harvest treatment of plant-derived food products from producer to consumer/processor to advance the production of high-quality foods.
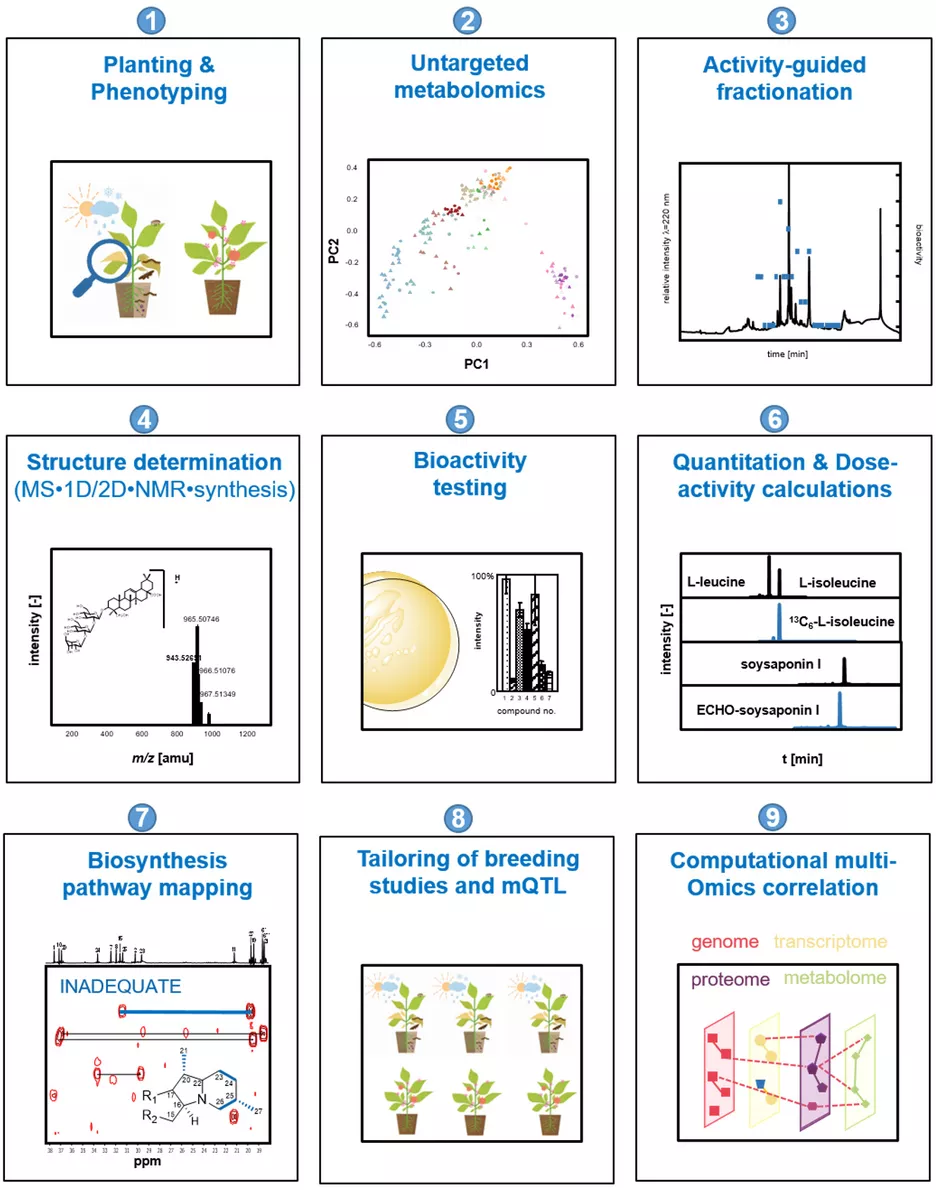
- Planting and phenotyping: The combination of phenotyping and metabolomics may provide insight into stress-induced metabolome alterations and may help to identify and quantify changes in the spectrum of key metabolites in quantitatively resistant versus susceptible genotypes, varieties, mutants, or phenotypes. Therefore, the planting of stressed and non-stressed plant genotypes in the field or greenhouse under defined and controlled conditions is indispensable. Moreover, the phenotyping of healthy and diseased plants is performed under state-of-the-art protocols (depending on stress application and plant material).
- Characterization of metabolome alterations by means of untargeted metabolomics to identify marker compounds: To determine which marker compounds are up- or downregulated and which biosynthetic pathways are influenced by abiotic or biotic stress challenges or both, stressed and non-stressed plant material samples are comparatively characterized for their low molecular weight metabolites. The following non-targeted metabolomics techniques are used: (i) HILIC/RP-UHPLC-TOF-MS, (ii) GCxGC-ToF-MS, (iii) DOLC NMR profiling, or both (ii) and (iii). First, all samples are extracted using solvents of different polarities, such as pentane, dichloromethane, ethyl acetate, methanol, a mixture of methanol and water, or water. To identify known metabolites, signal lists (NMR) and peak lists (MS) containing retention times, retention indices (RI), and high-resolution precursor and fragment m/z are compared to in-house or public databases or both (e.g., HMDB, Metlin, PlantCyc, Plant Metabolic Network, KEGG, RIKEN, NIST). Features that lack a positive database hit are identified through comparison with in-house or public databases, comparison of MS/MS spectra and retention times of commercially available or synthesized references, ion mobility analyses of collision cross-sections, and structural similarity networks. Bioinformatics-assisted marker molecule selection, followed by chromatographic purification of unknown marker molecules, reveal the chemical structures of up- or downregulated phytochemicals by means of structure determination experiments, as described in Step 4. The bioactivity of marker compounds is tested in Step 5.
- Activity-guided fractionation to identify bioactives (phytoalexins/phytoanticipins): To identify new phytoanticipins or phytoalexins or both that have potential for exploitation as marker substances, direct application in biological or organic cropping systems, or as chemical lead structures for nature-derived synthetic fungicides, several bioresponse-based discovery techniques are used. A combination of different bioassays, such as antifungal assays, and state-of-the-art chromatographic techniques are used to locate and isolate the bioactive phytochemicals. Volatiles are characterized via solvent extraction flavor evaporation (SAFE) and gas chromatographic techniques, whereas solid phase extraction (SPE), medium-pressure liquid chromatography (MPLC) and/or 1D/2D-high performance liquid chromatography (HPLC) sub-fractionation techniques combined with bioassays are used for the localization of non- or semi-volatile compounds. For structure identification (Step 4), bioactivity (Step 5), and quantitation experiments (Step 5), the non-volatile compounds are purified in sufficient quantities by MPLC and preparative or semi-preparative HPLC techniques or both or synthesized in purities higher than 98%.
- Chemical structure determination: The marker compounds (Step 2) and bioactives (Steps 2 and 3) are unequivocally identified by means of gas chromatography-mass spectrometry (GC-MS), ultra-high performance liquid chromatography-time of flight-mass spectrometry (UHPLC-TOF-MS), ultra-high performance liquid chromatography-time of flight-mass tandem spectrometry (UHPLC-MS/MS), ultraviolet visible maximum (UVmax), circular dichroism (CD), and one-dimensional/two-dimensional nuclear magnetic resonance (1D/2D-NMR) experiments, followed by independent chemical synthesis and co-chromatography experiments.
- Bioactivity of phytochemicals: The efficacy of the newly identified marker and bioactive compounds is tested using different bioassays, depending on the biotic stress factor. Secondary metabolites are known to have diverse biological and physio-chemical properties. Therefore, the substances from Steps 2 and 3 may be tested in additional assays for their potential use as antioxidants, antimicrobials, fungicides, antivirals, antitumorales, anti-inflammatories, antidiabetics, flavor-active compounds, color-active compounds, and so on.
- Quantitation of bioactives or marker compounds (targeted metabolomics): Once the marker compounds (Step 2) and bioactives (Steps 2 and 3) are identified (Step 4), individual (phyto)metabolites, phytohormones, and other signal molecules are quantitated in the target crop items by using 1D/2D GC-MS (volatiles), quantitative proton nuclear magnetic resonance (qHNMR; non-volatiles), and UHPLC-MS/MSMRM (non-volatiles and volatiles after derivatization techniques). To avoid work-up losses and matrix effects during GC-MS or LC-MS analyses, stable isotope-dilution analyses (SIDA) or the ECHO technique are used. In this technique, the stable, isotopically substituted analogues of the target compounds (2H- and/or 13C-labelled) are synthesized, and ECHO standards are isolated in purities higher than 98% in sufficient quantities by MPLC and preparative or semi-preparative HPLC techniques. All methods are validated. Recovery rates, inter- and intraday precision, limit of quantitation (LOQ), and limit of detection (LOD) are known.
- Biosynthesis pathway mapping: To gain additional insight into the biosynthetic pathways involved in the formation of phytoalexins or anticipines, cutting-edge technologies such as (i) isotopologue profilings, (ii) measurement of enzyme reactions, (iii) mutant developments, and (iv) artificial intelligence or bioinformatics tools (mapping genome or transcriptome-metabolome data) may be used. For example, incorporation experiments using stable-isotope-labelled precursors (e.g., 13C-labelled) or 13CO2 labelling followed by isotope analysis via GC-MS, LC-MS, and NMR analyses can be used to visualize dynamics in metabolic networks.
- Metabolomics-assisted tailoring of breeding studies: Targeted UHPLC-MS/MSMRM, qHNMR, or 1D/2D GC-MS quantitation studies help to identify and characterize primary and secondary metabolites in a large number of sample sets (e.g., samples of different genotypes, varieties, mutants, species, compartments, or development stages). Such studies also aid visualization of the impact of environmental conditions, beneficial pathogens, or abiotic and biotic stress factors on their concentrations. Thus, fast, robust, high-throughput, MS-based quantitation methods help to direct breeding for resistant and high-quality (crop) plants. These multiple methods help to direct understanding of breeding studies (e.g., for characterization or selection of genotypes and varieties or as a phenotyping tool for mQTL studies). They can also be used to optimize and understand post-harvest treatments.
- Bioinformatics analysis: genome/transcriptome - metabolome mapping: State-of-the-art machine learning and bioinformatics tools provided by open-source R or Python packages provide deeper insight into plants’ resistance and susceptibility mechanisms. For example, these tools may be used for classification and regression training or for random forest or Bayesian network analyses that include multiple omics and correlations (genomics, transcriptomics, or proteomics-metabolomics correlations). In addition, they can help to visualize genotype-dependent stress maps, to build stress databases, and to visualize the pan-metabolomes of crop plants.
SELECTED PUBLICATIONS:
Dawid, C.*; Hille, K. Functional metabolomics—a useful tool to characterize stress-induced metabolome alterations opening new avenues towards tailoring food crop quality. Agronomy 2018, 8(8), 138.
Kutschera, A.#; Dawid, C.#; Gisch, N.; Schmid, C.; Raasch, L.; Gerster, T.; Schäffer, M.; Smakowska-Luzan, E; Belkhadir, Y.; Vlot, C.; Chandler, C. E.; Schellenberger, R.; Schwudke, D.; Ernst, R.; Dorey, S.; Hückelhoven, R.; Hofmann, T.; Ranf, S. Bacterial medium-chain 3-hydroxy fatty acid metabolites trigger immunity in Arabidopsis plants. Science 2019, 364(6436), 178–181.
Baur, S.; Frank, O.; Hausladen, H.; Hückelhoven, R.; Hofmann, T.; Eisenreich, W.; Dawid, C.* Biosynthesis of α-solanine and α-chaconine in potato leaves (Solanum tuberosum L.) – A 13CO2 study. Food Chem. 2021, 365, 130461.
Schmid, C.; Sharma, S.; Stark, T.D.; Günzkofer, D.; Hofmann, T.F.; Ulrich, D.; Dunemann, F.; Nothnagel, T.; Dawid, C.* Influence of the abiotic stress conditions, waterlogging and drought, on the bitter sensometabolome as well as agronomical traits of six genotypes of Daucus carota. Foods 2021, 10(7), 1607.
Schmid, C.; Mittermeier-Kleßinger, V.; Peters, V. C. T.; Berger, F.; Kramler, M.; Heuberger, H.; Rinder, R.; Hofmann, T.; Gutjahr, C.; Dawid, C.* Quantitative mapping of flavor and pharmacologically active compounds in European licorice roots (Glycyrrhiza glabra L.) in response to growth conditions and arbuscular mycorrhiza symbiosis. J. Agric. Food Chem. 2021, 69(44), 13173–13189.
Dawid, C. Molecular characterization of stress-induced metabolome alterations in crops and model plants. Habilitation Thesis, TUM 2021.
Schellenberger, R.; Crouzet, J.; Nickzad, A.; Kutschera, A.; Gerster, T.; Borie, N.; Dawid, C., Cloutier, M.; Villaume, S.; Dhondt-Cordelier, S., Hubert, J.; Cordelier, S.; Mazeyrat-Gourbeyre, F.; Schmid, C.; Ongena, M., Renault, J.-H.; Haudrechy, A.; Hofmann, T.; Baillieul, F.; Clement, C.; Zipfel, C.; Gauthier, C.; Déziel, E.; Ranf, S.; Dorey, S. Bacterial rhamnolipids and their 3-hydroxyalkanoate precursors activate Arabidopsis innate immunity through two independent mechanisms. PNAS 2021, 118(39), e2101366118.
Mergner, J.; Frejno, M.; List, M.; Papacek, M.; Chen, X.; Chaudhary, A.; Samaras, P.; Richter, S.; Shikata, H.; Messerer, M.; Lang, M.; Altmann, S.; Cyprys, P.; Zolg, D. P.; Mathieson, T.; Bantscheff, M.; Hazarika, R. R.; Schmidt, T.; Dawid, C.; Dunkel, A.; Hofmann, T.; Sprunck, S.; Falter-Braun, P.; Johannes, F.; Mayer, K. F. X.; Jürgens, G.; Wilhelm, M.; Baumbach, J.; Grill, E.; Schneitz, K.; Schwechheimer, C.; Kuster, B. Mass-spectrometry-based draft of the Arabidopsis proteome. Nature 2020, 579, 409–414.
Das, D.; Paries, M.; Hobecker, K.; Gigl, M.; Dawid, C.; Lam, H.-M.; Zhang, J.; Chen, M.; Gutjahr, C. Phosphate starvation response transcription factors enable arbuscular mycorrhiza symbiosis. Nature Commun. 2022, 13, 477.
Chaudhary, A.; Chen, X.; Gao, J.; Leśniewska, B.; Hammerl, R.; Dawid, C.; Schneitz, K. The Arabidopsis receptor kinase STRUBBELIG regulates the response to cellulose deficiency. PLOS Genetics 2020, 16(1), e1008433.